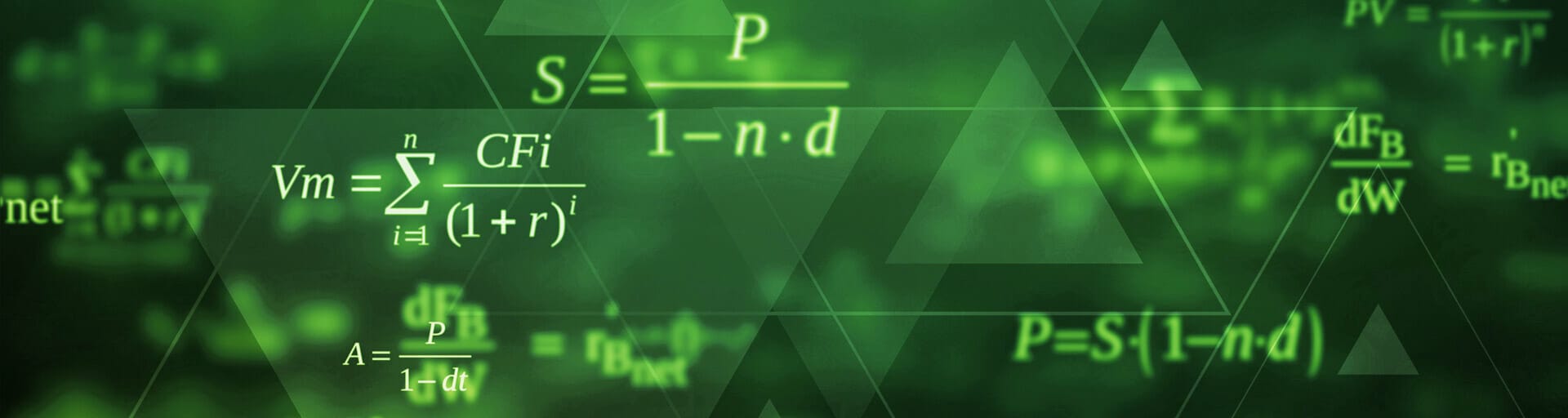
Research
The School of Complex Adaptive Systems has grown from these research groups and centers as we have sought to maintain open communication among groups with common interests, share research, and evolve a deeper understanding of the new science of complexity.
Our goals are:
- Provide an open forum for communication across disciplines.
- Encourage transdisciplinary collaborations to address seemingly intractable problems of complex systems at changing scales.
- Infuse education at all levels with complex adaptive systems thinking, while creating exciting new opportunities to train young and established scientists in the data integration and computational skills required now and in the long term for an evolving workforce.
Research Support
- Global Futures Office of Research Services – GFORS
GFORS‘s mission is to provide high-quality and comprehensive support of research activities across the “discovery space” of the Julie Ann Wrigley Global Futures Laboratory
ASU research network
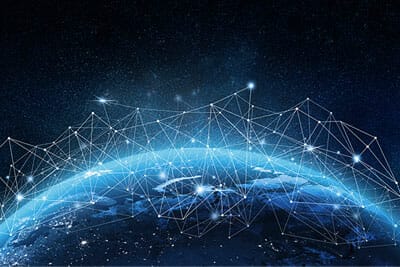
ASU-SFI Center for Biosocial Complex Systems
A partnership of Arizona State University and the Santa Fe Institute to discover the fundamental principles underlying complex systems.
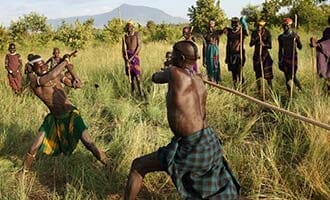
Adaptation, Behavior, Culture & Society Research Group
Bringing together scholars from across the social sciences and biological sciences who share an interest in using evolutionary tools, theories and insights to better understand how and why people behave as we do.
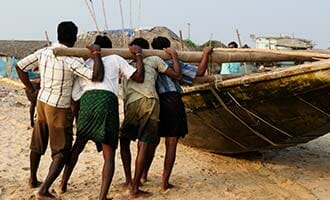
Center for Behavior, Institutions, and the Environment
A transdisciplinary endeavor to improve our understanding of effective governance of social-ecological systems.
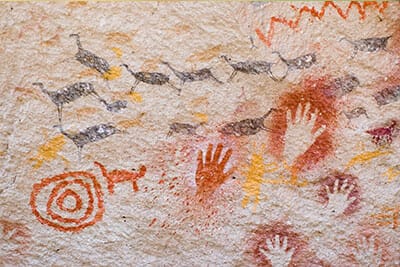
Center for Biology and Society
The Center for Biology and Society promotes research, education, and engagement related to study of the life sciences and their interconnections with society.
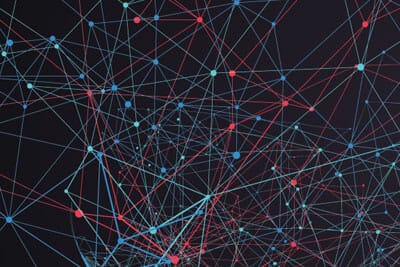
Complexity Economics Lab
The complexity economics lab at Arizona State University embraces a newly emerging paradigm to better understand economic processes.
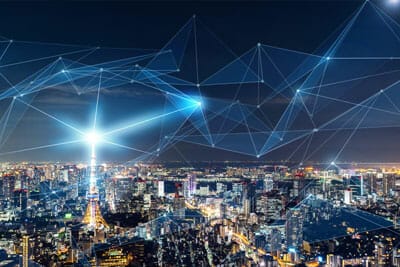
Complex Systems Research Group
The CSRG unites convergent research and expertise in complexity thinking and scientific computation to address urgent transdisciplinary questions about the future habitability of the planet and of sustainable social structures.
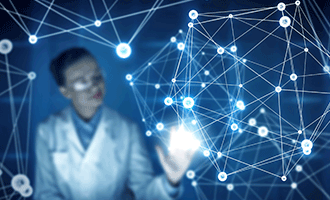
Data Science and Analytics
The Unit for Data Science and Analytics at ASU Library connects researchers university-wide to pursue research that leverages machine learning, data analytics, visual storytelling, network analysis, and text and data mining.
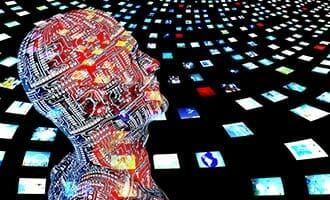
Decision Theater
The Decision Theater organizes researchers, policymakers and the business community to better understand and explore solutions to complex issues facing society.
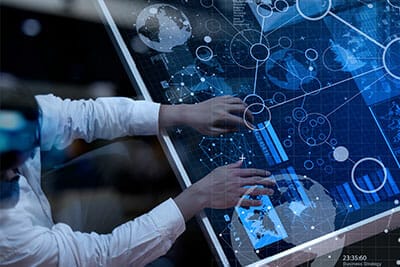
The Digital Innovation Group
The Digital Innovation Group pushes the boundaries of computational analysis in the humanities by developing and deploying innovative research software and analytic methods.
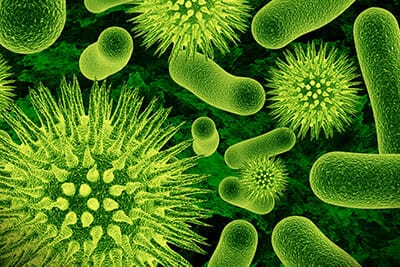
Center for Evolution & Medicine
Research that generates the Evidence Base as to why Evolutionary Medicine is important to achieve better health.
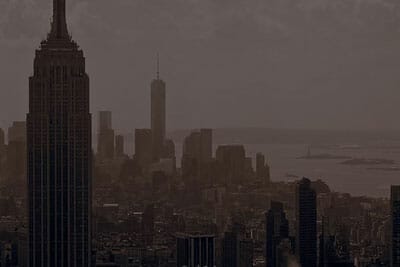
Regional Dynamics and Development
Uncovering the fundamental properties of regional systems and translating that knowledge into practical, data-driven tools to support decision makers.
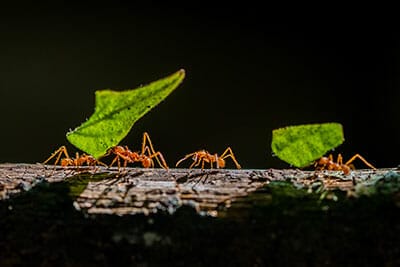
Social Insect Research Group
Studying the evolution and organization of insect societies from the level of the gene, up to their ecology and evolution.
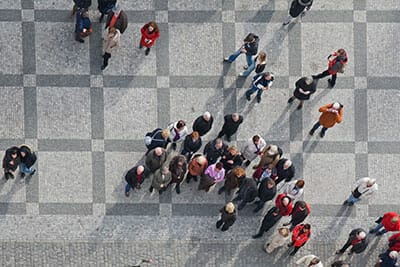
Center for Social Dynamics and Complexity
Interdisciplinary research to determine the algorithms of social dynamics across diverse contexts, ranging from genomes to entire organisms, and from individuals to cultures.
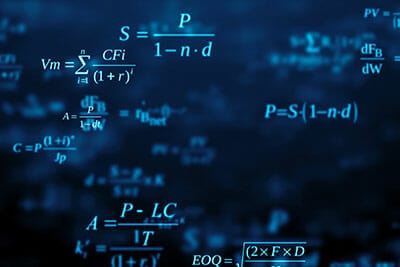
Simon A. Levin Mathematical, Computational and Modeling Sciences Center
Bridging the gap between the biological, mathematical, environmental, and social sciences.
International research network
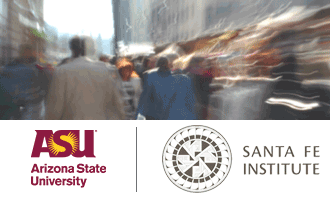
ASU-SFI Center for Biosocial Complex Systems
A partnership of Arizona State University and the Santa Fe Institute.
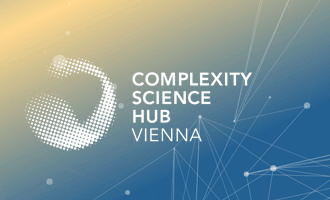
Complexity Science Hub Vienna
Where Big Data starts to make sense.
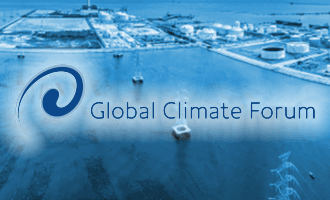
Global Climate Forum
The Global Climate Forum initiates and performs innovative research on climate change and related global challenges
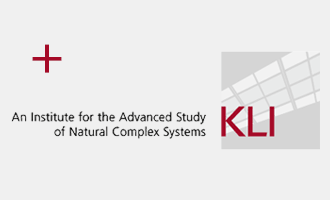
KLI: An Institute for the Advanced Study of Natural Complex Systems
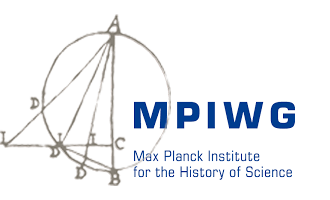
Max Planck Institute for the History of Science
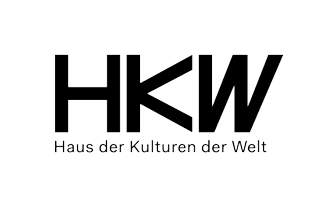
Haus der Kulturen der Welt
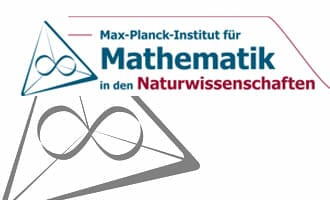